Authors
Linda Leon, Zbigniew Przasnyski, & Kala Chand Seal
Abstract
Most organizations use large and complex spreadsheets that are embedded in their mission-critical processes and are used for decision-making.
Identification of the various types of errors that can be present in these spreadsheets is, therefore, an important first step to creating controls that organizations can use to govern their spreadsheets. While a considerable amount of research on quantitative error taxonomies exists, there is comparatively little research concerning qualitative error taxonomies.
In this paper, we propose a taxonomy for categorizing qualitative errors in spreadsheet models that offers an exploratory framework for evaluating the quality of a spreadsheet model before it is released for use by others in the organization. The classification was developed based on types of qualitative errors identified in the literature and errors committed by end-users in developing a spreadsheet model for Panko's (1996) "Wall Problem."
A principal component analysis of the errors reveals four logical groupings thereby creating four categories of qualitative errors. The usability and limitations of the proposed taxonomy and areas for future research are discussed.
Sample
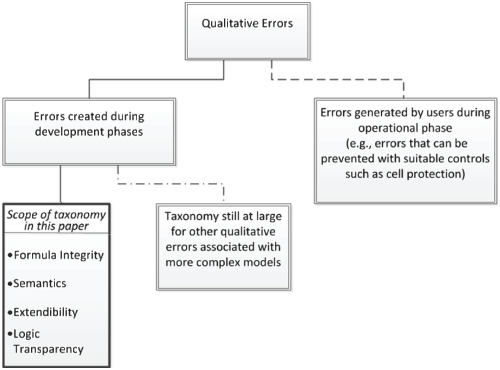
The taxonomy proposed in this paper provides four clearly defined categories for qualitative errors with examples of errors that can fall into each category. The categories are:
- Formula integrity. Focuses on latent errors associated with formulas used in the model. This category includes the two qualitative errors most commonly referred to in existing spreadsheet literature, the hard-coding or "jamming" of a value in a formula and the duplication of an input value.
- Semantics. Describes errors that create a distortion or an ambiguity in the identity and meaning of an input or output of the model. Common sources are poor documentation including incorrect cell formatting and labeling, lack of identification of assumptions and outputs, as well as the readability of the layout design.
- Logic transparency. Considers the vulnerability and the integrity of the output results and their interpretations given the transparency of the formula logic included in the model.
- Extendibility. Focuses on qualitative errors that place the model's integrity at risk when the model may need to be extended to make it operational.
Publication
2015, Journal of Organizational & End User Computing, January-March, pages 33-56
Full article
Introducing a taxonomy for classifying qualitative spreadsheet errors